Random Neurons Across Layers 1 & 2 of ResNet-50 with ImageNet-Val + Broden Probing Dataset
Neuron examples:
We have color-coded the neuron descriptions by whether we believed they were accurate, somewhat correct, or vague/imprecise.
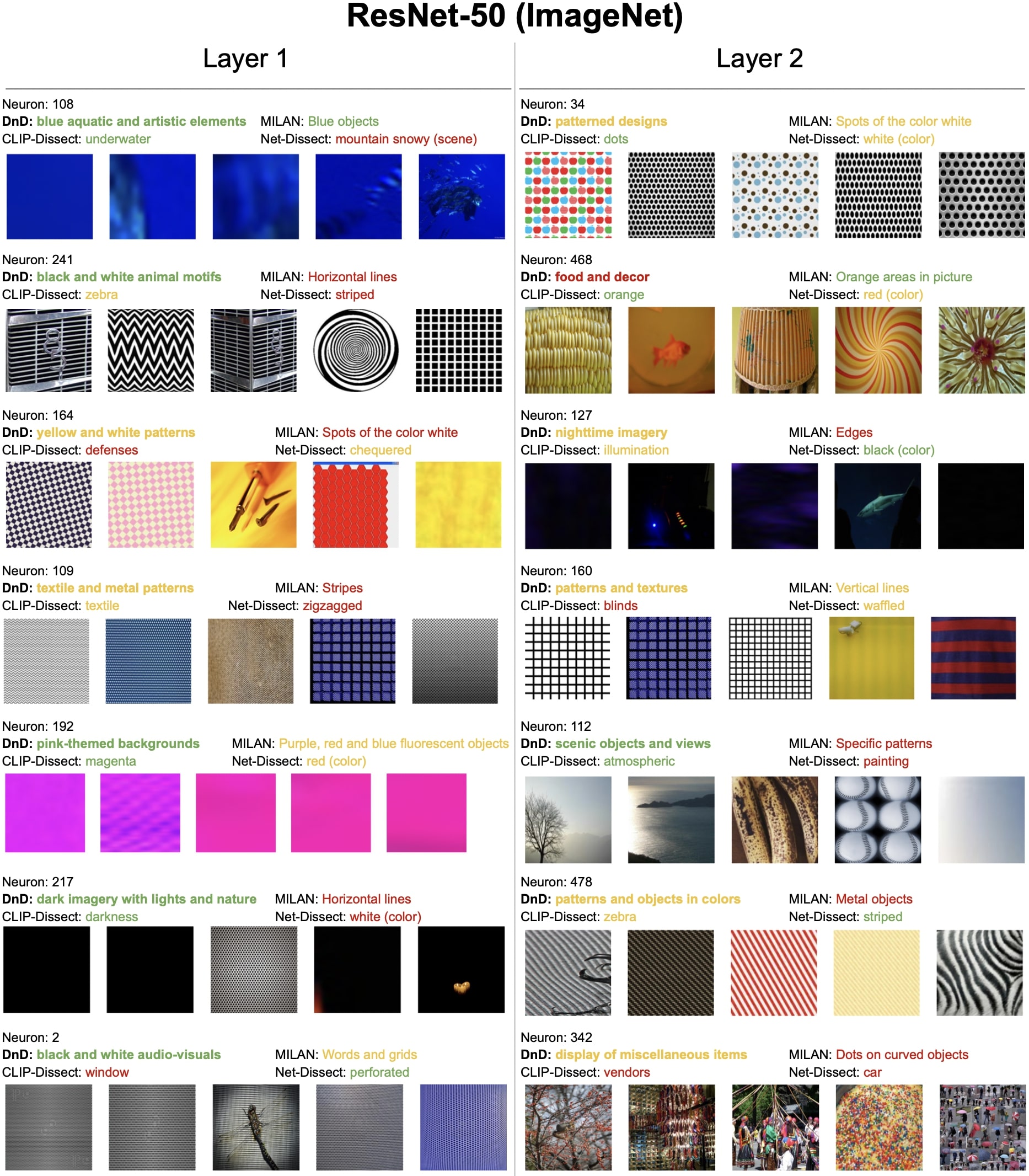
Neuron examples:
We have color-coded the neuron descriptions by whether we believed they were accurate, somewhat correct, or vague/imprecise.
Neuron examples:
We have color-coded the neuron descriptions by whether we believed they were accurate, somewhat correct, or vague/imprecise.
Neuron examples:
We have color-coded the neuron descriptions by whether we believed they were accurate, somewhat correct, or vague/imprecise.
Neuron examples:
We have color-coded the neuron descriptions by whether we believed they were accurate, somewhat correct, or vague/imprecise.
Neuron examples:
We have color-coded the neuron descriptions by whether we believed they were accurate, somewhat correct, or vague/imprecise.
Neuron examples:
We have color-coded the neuron descriptions by whether we believed they were accurate, somewhat correct, or vague/imprecise.
Neuron examples:
We have color-coded the neuron descriptions by whether we believed they were accurate, somewhat correct, or vague/imprecise.